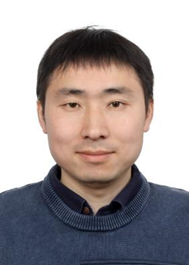
北京时间2020年7月25日周六上午10点,北京航空航天大学的汪待发副教授,博士生导师,将为大家讲解他们组去年发表的一篇脑机交互(BCI)的近红外文章。欢迎大家参加并参与讨论。
时间: 北京时间2020年8月29日周六上午10点
地点: https://zoom.com
房间号: 889 8026 7287
密码: 496792
ABSTRACT Functional near-infrared spectroscopy (fNIRS) has attracted much attention in brain-computer
interface (BCI) area due to its advantages of portability, robustness to electrical artifacts, etc. However, in practical applications, fNIRS-based BCI usually needs a labor-intensive and time-consuming training session (calibration procedure) to optimize the user-specifific neural spatial and temporal patterns for further classifification. Recently, studies revealed that neural spatial and temporal patterns extracted from user-specifific resting-state brain signals were closely related to those of his/her task data. In this study, we proposed a resting-state independent component analysis (RSICA) based spatial fifiltering algorithm aiming at extracting individual task-related spatial and temporal brain patterns from the resting-state data. Specififically, independent component analysis (ICA) was applied to extract different independent components (ICs) from resting-state fNIRS data. The ICs with their spatial fifilter weights maximally lateralized over the sensorimotor regions were regarded as most relevant to motor imagery. These spatial fifilters were used to spatially fifilter the multi-channel motor imagery task data for feature extraction. Based on 8-minute resting-state data and a small training dataset (20 trials) from 10 participants, the proposed RSICA algorithm achieved an approximately 7% increase in left vs. right hand motor imagery classifification accuracy, as compared to the conventional common spatial pattern (CSP)-based and shrinkage algorithms (69.8±12.1%, 63.3±10.3% and 63.4±11.8%, respectively). For acquiring a similar level of classifification accuracy (i.e. 70%), the number of training data required could be reduced from 36 trials (CSP) to 22 trials (RSICA). As a relatively small training set is required to obtain a satisfactory performance, training burden is signifificantly reduced by RSICA, which might be useful for developing practical fNIRS-based motor imagery BCIs.